If you were ever assigned a mandatory essay (or ten) in high school, you can probably remember the anguish that went into writing that first sentence. I remember spending hours watching the cursor blink at me from an empty word document as I combed desperately through my notes for an engaging “hook sentence” to “grab my reader”. And even as I have progressed from writing high school essays to postgrad theses and research papers, that first sentence always remains just as elusive.
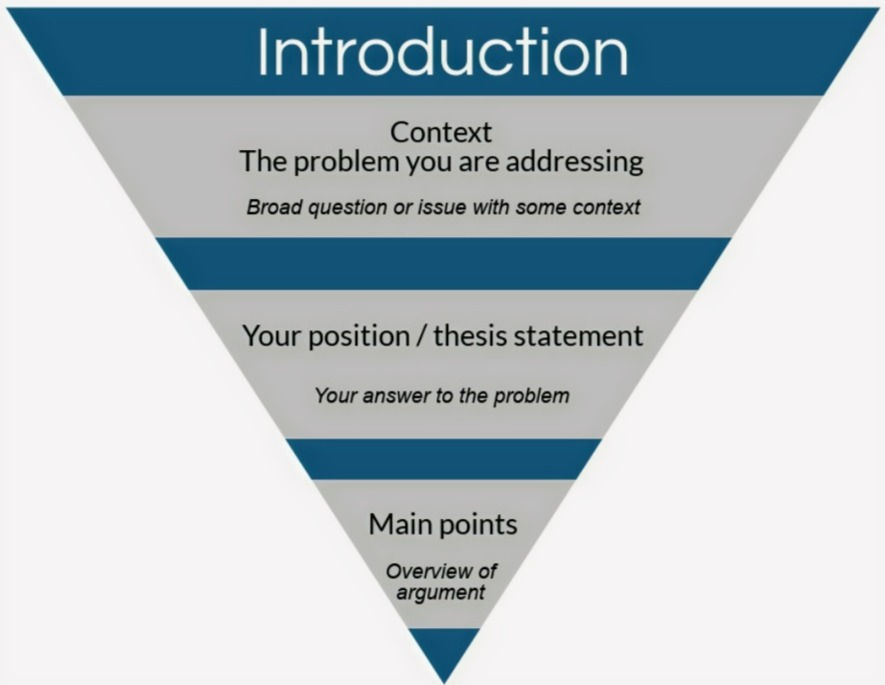
Luckily for me, I have learned that scientists have a trick up their sleeve when it comes to writing articles. In academia, introductions serve a specific purpose and can be constructed in a formulaic way. The details might differ between disciplines, but everyone agrees on the importance of establishing context in your first few sentences.
This is particularly essential in biomedical research, especially if your topic of study is some plant or bacteria species with an unintelligible Latin name. For example, I just came across an article called, “Advances in Microbial Production of Polyhydroxyalkanoates”. If this paper doesn’t explain what a Polyhydroxyalkanoate is and why it matters within the first two sentences, I must confess that I might not make it beyond that point. Molecular scientists have to explain the broader context behind their otherwise arbitrary-sounding molecule of interest, provide readers outside of their field with some essential pieces of background info, and illustrate the significance of their work in the real world. That's why, in many fields, there is an unspoken rule to include a clear definition for your topic of study at the beginning of your paper. And the field of autism research is no exception.
In their recent article entitled "Rethinking Our Concepts and Assumptions About Autism", Lombardi and Mandelli begin by noting that: “Nearly every article on autism tends to start off in the same way. “Autism is <insert paraphrased DSM definition, or core symptom domains here>”. I can attest that this is certainly true of almost every article I have read during my postgrad research – including, in my quest towards proper academic writing practices, many of my own papers and assignments. But our understanding of autism is undergoing a fundamental shift with respect to its diagnostic criteria and underlying complexity.
What was once understood as a disorder marked by a defined set of symptoms is now recognized as a different neurotype with presentations that are much more heterogenous, dynamic and context-dependent. Clinicians are realizing that many diagnostic tools don't sufficiently encompass the variance across the spectrum – and this is particularly true for populations that have historically been under-represented in research. There is no question that our current diagnostic guidelines are useful in a clinical context and facilitate access to essential resources and accommodations. But, as Lombardi and Mandelli highlight, the “definition” for autism that is so widely included in academic literature as an “objective medical diagnosis” doesn’t always reflect the true context of this research.
The ongoing debates about theoretical conceptualizations, while essential to drive progress in the clinical and sociological context, are often considered to be outside the scope of biomedical research. Scientists have to define their topic of study somehow, and it makes sense to refer to well-established clinical diagnoses for this purpose. But the heterogeneity of autism is reflected on the molecular level, and this has important implications for molecular research. We know that existing clinical guidelines are based on the presentation of external behaviours, which can seldom provide insight into underlying molecular processes. So it is critical for molecular scientists to consider the tangible implications of using these clinical criteria to fundamentally define the parameters of our work. In my previous post, I discussed some of the central questions surrounding the aims and objectives of molecular research that must also be carefully considered in this context. Here, I will explore how the inherent heterogeneity of autism presents some significant limitations - but also unique opportunities - for molecular research, and how this informs our own research in a South African context.
First, I think it is useful to give you an idea of how molecular studies usually work in a different context. A typical molecular workflow might look like this:
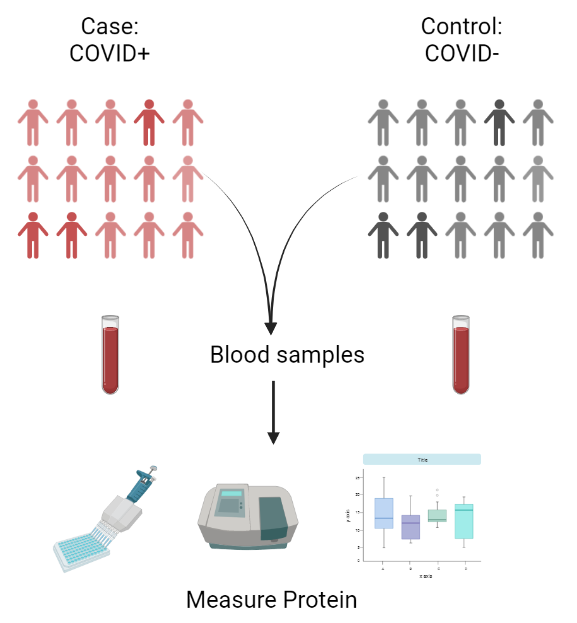
Researchers start by collecting a group of individuals – a “cohort” – that is categorized according to their phenotype. In COVID-19 research, for example, researchers might do a quick PCR test to find out who has the virus (a “case") and who doesn’t (a “control").
They might measure the levels of a particular immune protein in blood samples from all participants.
Finally, they will run a statistical analysis to decide whether the levels of that protein are significantly changed by COVID-19 infection.
Now imagine how we might try to apply this workflow in autism research. Let us say that a researcher gathers 50 individuals to take part in their study - half of them with and half without a clinical autism diagnosis. The question is, how do you define a “case” with so many different presentations of autism, and so many comorbidities, that range across such a vast spectrum and aren’t always identified in a clinical context? How do you define a “control” when there is no single definition for “neurotypical"? And how can we decide what protein or molecular target to measure, when each individual autism presentation might have a different molecular origin?
As I mentioned before, diagnostic criteria can be far removed from underlying biological processes and internal lived experiences. This means that some individuals in the "control" group might actually be autistic individuals who have remained undiagnosed or misdiagnosed due to a lack of access to healthcare, high levels of masking or compensation, or a presentation that is not yet reliably captured using current diagnostic tools. Conversely, some individuals with an autism diagnosis could have a range of hidden comorbidities, or they could be misdiagnosed due to a different neurological or psychological condition. And, since external behaviours don't really tell us enough to choose a single molecular target, many autism studies have to start with a whole-genome approach. This gives us an underlying molecular signature created by thousands of genes and interactions between them, which will be different in every single individual irrespective of diagnostic status.

Case/control cohort without stringent phenotyping: Each different colour represents a different molecular signature comprised of tens of thousands of genes and interactions between them. Individuals with autism are depicted in varying colours, non-autistic individuals are depicted in shades of grey. You can see that there are many different colours in each group, and several individuals in both groups have been miscategorized.
The figure above depicts each of these different molecular signatures as a different colour. Individuals in both groups have a range of different colours. So, how do we decide if one group is significantly different to the other as a whole? Now, there is a whole field of research dedicated solely to this question of statistical significance, but I am just here to give you the general idea. (To the statisticians and bioinformaticians - you might want to skip this paragraph for the sake of your own psychological well-being). When we try to identify a distinct molecular signature associated with autism, scientists essentially “mix” all the colours in the "control" group together to get the average molecular signal for the group. Then, we do the same for all the individuals in the "case" group, and see if this generates a signal with a different colour. In the example above, the controls, who are mostly grey, will give you a grey average signal. But there are so many different colours are in the "case" group, that when we mix them all together, they also blend into a murky grey that is indistinguishable from the control signal! This is what happens when molecular studies don’t conduct rigorous cohort phenotyping.
In order to navigate this complexity, researchers have to try and minimize the variation within each group so that a distinct molecular signature can be isolated. Our recent papers (listed here) investigated a novel molecular signature associated with autism in a South African cohort. In our studies, we used something called “age and gender-matched controls”, meaning that all individuals in our cohort were the same age and gender to limit variation due to these factors. Then, we used two layers of phenotyping to distinguish the “cases” from the “controls”. Like many studies, our first layer of phenotyping was based in the presence or absence of a clinical autism diagnosis. Second, we used a specific diagnostic tool called the ADOS2 to screen each individual in both the case and control group. Participants with an autism diagnosis who did not meet all ADOS2 criteria were excluded; as were individuals in the “control” group who did meet these criteria. This ultimately produced a cohort comprising of a specific subset of autistic individuals and a specific subset of controls, where each group had much lower levels of within-group variation.

Case/control cohort with stringent phenotyping. We started off with many different colours in both groups. Filtering each group using the ADOS2 helped to decrease the number of miscategorized individuals, and selected for a specific "blue" autism presentation. This helped us to isolate a molecular signature that was associated with autism in our cohort.
Importantly, this approach is only rooted in a logistical necessity to reduce variation in the context of molecular research. This is distinct from the utility of certain diagnostic tools in a clinical context. The phenotyping approach we used does not reflect an assumption that all individuals with autism would meet ADOS2 criteria, nor that external behaviours can be used to differentiate different molecular signatures. Rather, we know that many autistic individuals remain undiagnosed, particularly in a context where health care is not readily accessible, which is why we cannot solely rely on clinical diagnoses. And while external behaviours are not specifically linked to certain molecular processes, the hope is that by selecting a specific subgroup of individuals with similar presentations, we might be able to reduce the underlying variation in both groups enough to be able to isolate a meaningful molecular association.
That said, it is also important to recognize that this work reflects some long-term systemic challenges in the field. The use of pre-existing diagnostic tools in molecular research means that much of the current literature is not necessarily generalizable to many previously under-studied populations. This is a self-perpetuating cycle; diagnostic tools are generated based on homogenous populations, and these same tools are subsequently used to define the parameters of autism research. For example, our cohort was comprised of male children between 6 and 12 years old based on i) the fact that fewer females with autism are diagnosed and ii) the shortage of clinical networks that provide resources to autistic individuals beyond primary and high school (see Pillay et al, 2020). While our parameters were dictated by logistics, which I think many researchers can understand, this work still contributes to a field that has predominantly studied autism in males and young children. On a global scale, this is a systemic problem that has translated into a critical lack of accommodations or resources for adults and females with autism, and too frequently culminates in negative clinical outcomes.

At the same time, molecular autism research remains dominated by studies from the countries in the global north, like the UK and the USA. We have to start somewhere to try and bridge the gap, because African populations represent one of the most understudied populations in the field. This is partly reflective of a significant shortage of qualified diagnosticians and established clinical networks, and poor public awareness about autism. Not to mention the cost of molecular research when you are paying in Rands for specialized reagents or instruments from overseas! Expanding molecular research to include understudied populations is an important step in working towards a field that is more inclusive and internationally relevant. In fact, this is a particularly promising avenue for future research precisely because of autism's vast underlying heterogeneity.
From a molecular perspective, South African populations have very a different genetic history to populations in other areas of the world. Intuitively, many of us understand that South African populations have different genetic signatures to populations from Europe, for example. This is how companies like 23andMe can use your DNA sequences to figure out your ancestry. So, it follows that South African people with autism might also have very different molecular signatures compared to the autism cohorts that have been studied in the global north.
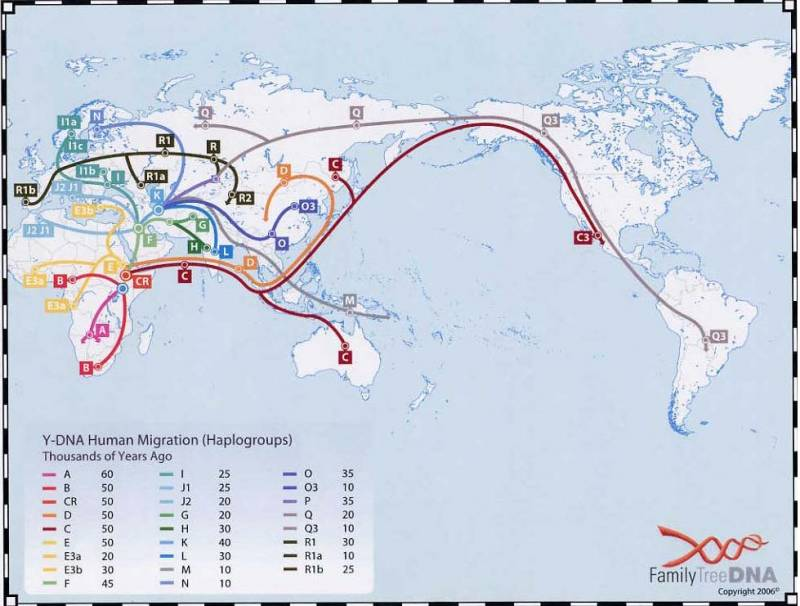
In fact, you might know that human history can be traced back to Africa, meaning that our genetic lineage is incredibly unique and rich in variation. Our studies don't actually focus on genetic sequences – we use approaches called epigenomics and transcriptomics, which reflect more dynamic molecular mechanisms at a single snapshot in time. But the genetic variation inherent to South African populations will likely be reflected by novel variation at the level of these dynamic processes.
What’s more, we know that many of the disparate molecular signatures implicated in autism tend to converge on a smaller number of downstream biological processes. This is why expanding current research to include historically under-represented populations could help us to find new molecular signatures that have not yet been found in other populations – and why this signature could tell us something about the convergent processes that are shared across many presentations of autism.
This puts molecular researchers, particularly in the global south, in a bit of a predicament. As our understanding of molecular autism aetiology improves, we are increasingly aware of the need to expand current research to include understudied populations, but also of the limitations of diagnostic tools that make our research possible. While there is no simple resolution, I think that Lombadi and Mandelli summed it up best with this well-known statistical axiom: “All Models are Wrong – but Some are Useful”. In science, "models” usually refer to cells, mice, or equations, which are used to ask specific molecular questions with limited scope in a simple context. But I think most cohort studies in autism research can be viewed the same way: as a potentially useful model.
These studies, by necessity, over-rely on medical models of disability, limited measurements of behavior, and flawed indicators of functioning. The consensus is that much previous research focused on a small homogenous subset of the autism spectrum. And crucially, in the absence of a comprehensive definition for autism, all molecular studies rely on one-dimensional, reductionistic diagnostic criteria to separate "case" from "control" - even though these criteria are themselves grounded in evolving models that are still being refined by clinicians and sociologists in an ongoing attempt to conceptualize autism and neurodiversity. All of these studies are wrong in that they fail to encompass evolving understandings of autism, and its underlying heterogeneity. But all of these studies are also useful in that they provide insight into disparate molecular signatures and convergent molecular mechanisms that underly autism aetiology.
I think it is still important to grapple with ways in which molecular research could be less wrong and more useful – that is to say, not so far removed from the lived experiences of autistic individuals and more translatable in a sociological and clinical context. But molecular research is complicated and it takes time. Science will be wrong sometimes - in fact, I have discovered that this appears to be a big part of the process. My own post-grad research journey reads like an instalment of Lemony Snicket's "A Series of Unfortunate Events". So maybe being right is less important than mitigating the potential for harm when we are wrong. Molecular research may be logistically tied to one-dimensional definitions of autism in terms of experimental design, but insight into the intricacies of internal autistic experiences can still fundamentally change the way we conduct, contextualize and communicate our work.
The field is already being reshaped by participatory research practices and a more biopsychosocial approach to disability. What's more, all molecular scientists will agree that understanding the shortcomings of our existing models is essential to make sense of our findings. In fact, our work makes us acutely aware of the limitations of current clinical criteria to capture the complexities we encounter in our studies. None of what I have discussed here is likely to be news to researchers in the field - when articles use the clinical "definition" for autism, most of this goes without saying. But if things are never said, they also aren't communicated. And I think that molecular research has the most potential to do harm when it is lost in translation.
Ultimately, my goal with this post was just to communicate some of the uncertainty and nuance behind the how and why of our research methodologies that can't always be converted into a neat "context sentence" at the beginning of a paper. Unfortunately, to the great chagrin of my internal academic rulebook, I don't have a clear concluding statement for you either. Instead, I will leave you with one of my favourite idioms, that has felt increasingly relevant as I try to reconcile the dualities of doing molecular research in this context:
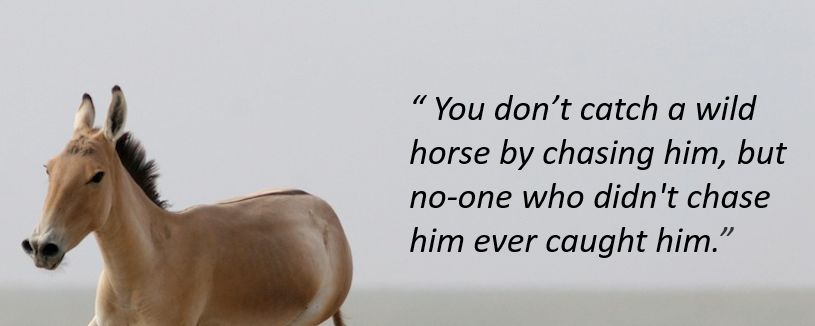
Read Part One Learn more about our research.
Comments